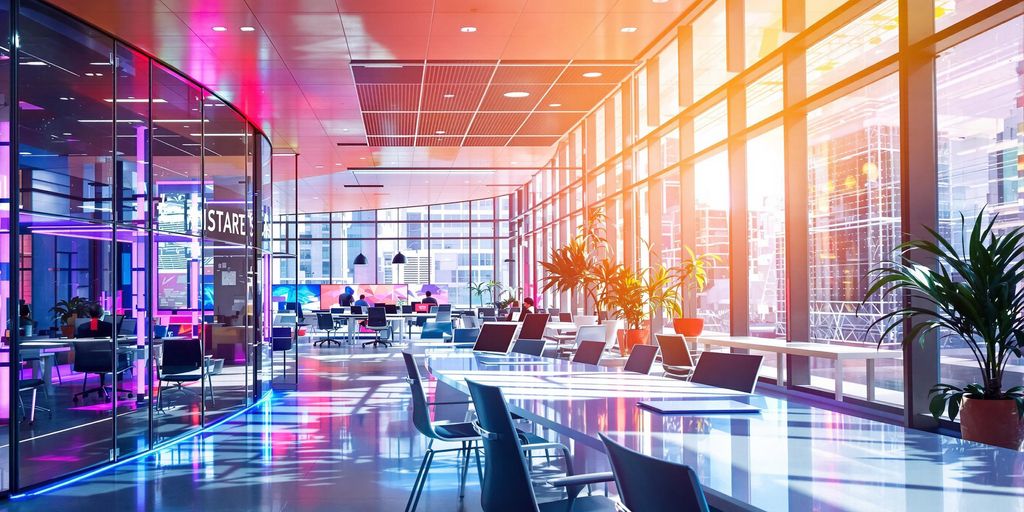
In today’s fast-paced business world, staying relevant is key to success. Relevance AI is changing the game by helping organizations make smarter decisions, improve operations, and connect better with customers. This article explores how businesses can harness Relevance AI to enhance their strategies and drive growth.
Key Takeaways
- Relevance AI helps businesses make informed decisions based on data-driven insights.
- Integrating AI into daily operations can streamline workflows and boost efficiency.
- Training teams on AI tools fosters innovation and collaboration across departments.
- Personalized experiences powered by AI lead to higher customer satisfaction.
- Establishing strong data governance is essential for trust in AI systems.
Harnessing Relevance AI For Strategic Decision-Making
Understanding Data-Driven Insights
Okay, so you’ve got all this data, right? But what does it mean? That’s where Relevance AI comes in. It’s not just about collecting numbers; it’s about turning those numbers into actual, useful insights. Think of it like this: you’re trying to find a specific book in a massive library. Relevance AI is the librarian who knows exactly where to look and can tell you why that book is important to you. It helps you understand the story the data is trying to tell, so you can make smarter calls. For example, you can use strategic decision-making models to improve your business.
Aligning AI with Business Goals
The biggest mistake companies make with AI is treating it like a shiny new toy instead of a tool. It’s gotta serve a purpose, and that purpose needs to be directly tied to your business goals. Are you trying to increase sales? Improve customer retention? Streamline operations? Whatever it is, your AI strategy needs to be laser-focused on achieving those specific objectives. Otherwise, you’re just wasting time and money. It’s like trying to build a house without a blueprint – you might end up with something, but it probably won’t be what you wanted.
Creating a Feedback Loop for Continuous Improvement
AI isn’t a set-it-and-forget-it kind of thing. It’s constantly learning and evolving, and your strategy needs to reflect that. That means setting up a system for monitoring performance, gathering feedback, and making adjustments as needed. Think of it like training a dog. You don’t just teach it a trick once and expect it to perform perfectly every time. You need to provide ongoing reinforcement and correct any mistakes along the way. The same goes for AI. You need to constantly refine your approach to ensure it’s delivering the best possible results.
It’s important to remember that AI is a tool, not a magic bullet. It can be incredibly powerful, but it’s only as good as the data you feed it and the strategy you use to guide it. By focusing on relevance and aligning your AI efforts with your business goals, you can unlock its full potential and drive real, measurable results.
Integrating Relevance AI Into Business Operations
Transforming Daily Workflows
Relevance AI isn’t just about fancy algorithms; it’s about changing how things get done every day. Think of it as injecting intelligence into the mundane. Instead of employees spending hours on repetitive tasks, AI can automate those processes, freeing them up for more strategic work. For example, AI can automatically categorize and route customer emails, reducing response times and improving customer satisfaction. It can also automate data entry, reducing errors and improving data accuracy. This shift requires a careful assessment of current workflows to identify areas ripe for AI intervention. It’s not about replacing people, but about augmenting their abilities.
Enhancing Operational Efficiency
AI can really boost how efficiently a business runs. It’s not just about doing things faster, but also smarter. For example, predictive maintenance uses AI to analyze data from equipment and predict when it might fail. This allows businesses to schedule maintenance proactively, reducing downtime and saving money. AI can also optimize supply chains, predicting demand and adjusting inventory levels accordingly. This reduces waste and ensures that businesses always have the products they need on hand. The key is to identify bottlenecks and inefficiencies in current operations and then use AI to address them. This is where business research comes in handy.
Here’s a simple example of how AI can improve efficiency in a warehouse:
Process | Without AI | With AI |
---|---|---|
Inventory Check | Manual, time-consuming, prone to errors | Automated, real-time, accurate |
Order Fulfillment | Manual picking and packing | AI-optimized routing and automated packing |
Shipping | Manual label creation and scheduling | Automated label creation and optimized routes |
Measuring Impact Through KPIs
It’s important to track the impact of Relevance AI on business operations. This means setting clear Key Performance Indicators (KPIs) before implementing AI and then monitoring those KPIs over time. For example, if the goal is to improve customer satisfaction, then the KPI might be customer satisfaction scores. If the goal is to reduce costs, then the KPI might be operating expenses. It’s also important to track the impact of AI on employee productivity and engagement. Are employees more productive and engaged after AI is implemented? If not, then something needs to change. It’s a good idea to have a baseline before implementing AI, so you can compare the results after implementation. This helps to ensure that AI is actually delivering the desired results.
Measuring the impact of AI through KPIs is not just about numbers; it’s about understanding the story behind the numbers. Are we achieving our goals? Are we improving the lives of our employees and customers? Are we building a more sustainable and resilient business? These are the questions that KPIs can help us answer.
Empowering Teams With Relevance AI Tools
It’s not enough to just have AI; you need to make sure your people can actually use it. That means giving them the right tools and the right training. Think of it like buying a fancy new oven but not teaching anyone how to bake. What’s the point?
Training for AI Proficiency
Okay, so you’ve got some AI tools. Great! Now, how do you get your team up to speed? It’s all about training, and not just the basic stuff. We’re talking about really getting into the nitty-gritty so people feel comfortable using these tools every day. It’s about bridging the skills gap that often exists between engineers and data scientists.
Here’s a few things to consider:
- Start with the basics: Not everyone needs to be a data scientist, but everyone should understand the fundamentals of AI and how it can be applied to their work.
- Hands-on workshops: Theory is great, but practical experience is better. Get your team working with real data and real-world problems.
- Ongoing support: AI is constantly evolving, so training shouldn’t be a one-time thing. Provide ongoing support and resources to help your team stay up-to-date.
Fostering a Culture of Innovation
It’s not just about learning how to use the tools; it’s about encouraging people to experiment and find new ways to apply AI. A culture of innovation is key to getting the most out of your AI investments.
If people are afraid to fail, they’re not going to try anything new. Create an environment where it’s okay to experiment, even if it means making mistakes along the way. That’s how you discover new and innovative uses for AI.
Encouraging Cross-Functional Collaboration
AI isn’t just for the tech team. It’s a tool that can be used by everyone, from sales and marketing to customer service and finance. But to make that happen, you need to break down the silos and encourage cross-functional collaboration. Think about how AI-powered chatbots can improve customer satisfaction metrics.
Here’s how to make it happen:
- Create cross-functional teams: Bring together people from different departments to work on AI projects.
- Share knowledge and best practices: Make sure everyone has access to the same information and resources.
- Celebrate successes: When a team achieves a success with AI, make sure to celebrate it and share the learnings with the rest of the organization.
Department | AI Application | Benefit |
---|---|---|
Sales | Lead scoring | Increased conversion rates |
Marketing | Personalized email campaigns | Higher engagement and click-through rates |
Customer Service | Chatbot support | Reduced wait times and improved satisfaction |
Driving Customer Engagement Through Relevance AI
Relevance AI is changing how businesses connect with their customers. It’s not just about sending messages; it’s about sending the right messages to the right people at the right time. This can lead to happier customers and better business results. Let’s look at how Relevance AI can make a difference.
Personalized Customer Experiences
Customers now expect personalized experiences. Generic marketing just doesn’t cut it anymore. Relevance AI can analyze customer data to understand individual preferences and behaviors. This allows businesses to create tailored experiences, from personalized product recommendations to customized content. Think about it: a clothing retailer could suggest items based on a customer’s past purchases and browsing history. Or a streaming service could recommend shows based on what a user has already watched and liked. This level of personalization makes customers feel valued and understood, which can lead to increased loyalty.
Utilizing AI-Powered Chatbots
AI-powered chatbots are becoming increasingly common on websites and apps. They can provide instant support, answer questions, and even guide customers through the purchasing process. The cool thing is that these chatbots are getting smarter all the time. They can learn from past interactions and use that knowledge to provide even better service in the future. This not only improves the customer experience but also frees up human agents to focus on more complex issues. It’s a win-win.
Here’s a quick look at the benefits of using AI chatbots:
- 24/7 availability
- Instant responses
- Reduced wait times
- Cost savings
Improving Customer Satisfaction Metrics
Ultimately, the goal of using Relevance AI is to improve customer satisfaction. But how do you measure that? There are several key metrics that businesses can track, including:
- Net Promoter Score (NPS): Measures customer loyalty and willingness to recommend your business.
- Customer Satisfaction Score (CSAT): Measures how satisfied customers are with a specific interaction or experience.
- Customer Effort Score (CES): Measures how much effort customers have to put in to resolve an issue.
By tracking these metrics, businesses can see how Relevance AI is impacting customer satisfaction and make adjustments as needed. For example, if you notice that your NPS is declining, you might need to re-evaluate your personalization strategy or improve the performance of your AI-powered chatbots. Using Customer.io can help you design and oversee personalized communications with your customers, enhancing engagement and marketing effectiveness.
Relevance AI isn’t just a technology; it’s a way of thinking about customer engagement. It’s about putting the customer at the center of everything you do and using data to create experiences that are relevant, valuable, and personalized. When you do that, you’re not just improving customer satisfaction; you’re building stronger relationships and driving long-term business success.
Building Trust in Data for Relevance AI
Data is the fuel that makes Relevance AI work. If you don’t trust your data, you won’t trust the AI, and that’s a problem. It’s like trying to bake a cake with bad ingredients – the result just won’t be good. So, how do you build that trust? It starts with getting serious about how you handle your data.
Establishing Data Governance
Think of data governance as the rules of the road for your data. It’s about setting up policies and procedures to manage data properly. A solid data governance framework ensures data is accurate, consistent, and compliant with regulations. Without it, you’re driving blind. This includes defining who’s responsible for what, how data is collected, stored, and used, and how to handle any issues that come up. It’s not the most exciting stuff, but it’s super important. A data strategy is key to success.
Ensuring Data Quality and Security
Data quality is all about making sure your data is accurate, complete, and reliable. Garbage in, garbage out, right? You need to validate and cleanse your data regularly. This might involve fixing errors, removing duplicates, and filling in missing information. Security is just as important. You need to protect your data from unauthorized access and breaches. This means implementing strong security measures like encryption and access controls. Think of it like this:
- Regular data audits to spot problems early.
- Data validation processes to catch errors as they happen.
- Employee training on data security best practices.
Data quality and security aren’t just IT issues; they’re everyone’s responsibility. When everyone understands the importance of data integrity and security protocols, it creates a culture of trust and accountability around data.
Creating a Unified Data Strategy
A unified data strategy means bringing all your data together into one place. This could be a data warehouse, a data lake, or some other centralized system. The goal is to have a single source of truth for all your data. This makes it easier to analyze, easier to manage, and easier to trust. It also means breaking down data silos and encouraging collaboration across different departments. A unified data strategy also involves data foundations for AI.
Data Source | Current Status | Integration Plan |
---|---|---|
CRM | Isolated | Integrate into DWH |
Marketing | Inconsistent | Standardize data |
Sales | Outdated | Real-time updates |
Scaling Relevance AI Solutions Across the Organization
Identifying Key Areas for AI Implementation
Okay, so you’ve got some Relevance AI solutions that are working well. Great! Now, how do you get them everywhere? It starts with figuring out where AI can make the biggest splash. Don’t just throw AI at every problem; be strategic. Think about the areas where AI can really move the needle, like improving customer service, streamlining operations, or even sparking new product avenues. Prioritize those areas first. It’s about impact, not just implementation.
Leveraging Cloud-Based Infrastructure
Let’s be real: scaling AI without the cloud is like trying to run a marathon in flip-flops. Cloud infrastructure is pretty much essential for handling the data and processing power that AI needs. Cloud platforms offer the flexibility and scalability to grow your AI solutions without breaking the bank. Plus, they often come with built-in AI services that can make your life a whole lot easier. Think about it: no more worrying about servers crashing or running out of storage. Just pure, unadulterated AI power.
Adopting MLOps for Scalability
MLOps? It’s basically DevOps, but for machine learning. It’s all about automating and streamlining the AI development and deployment process. Think of it as the secret sauce that lets you scale your AI solutions quickly and efficiently. With MLOps, you can:
- Automate model training and deployment.
- Monitor model performance in real-time.
- Easily roll back changes if something goes wrong.
- Ensure consistency across different environments.
Implementing MLOps isn’t just a nice-to-have; it’s a must-have if you’re serious about scaling your Relevance AI solutions. It helps you avoid the chaos and headaches that can come with managing complex AI deployments.
Here’s a simple table to illustrate the benefits:
Feature | Without MLOps | With MLOps |
---|---|---|
Deployment Speed | Slow | Fast |
Error Rate | High | Low |
Scalability | Limited | High |
Navigating Ethical Considerations in Relevance AI
It’s easy to get caught up in the excitement of new tech, but we can’t forget about the ethical side of things. With Relevance AI, this is super important. We’re dealing with data, predictions, and decisions that can seriously impact people’s lives. So, let’s talk about how to do it right.
Ensuring Fairness and Transparency
AI systems should be fair to everyone, regardless of their background. This means actively working to remove bias from your data and algorithms. It’s not enough to just hope your AI is fair; you need to test it, check it, and keep an eye on it. Think about how your AI might affect different groups of people, and make sure you’re not accidentally creating unfair outcomes. Transparency is also key. People should understand how your AI works and how it makes decisions. Black boxes aren’t acceptable when people’s lives are on the line. Consider using explainable AI techniques to make your models more understandable. For example, you can use product data to ensure fairness.
Addressing Privacy Concerns
Data privacy is a big deal, and it’s only getting bigger. You need to be super careful about how you collect, store, and use personal data. Make sure you’re following all the relevant laws and regulations, like GDPR or CCPA. But it’s not just about compliance; it’s about respecting people’s privacy. Consider using techniques like data anonymization and differential privacy to protect sensitive information. Get user consent before collecting data, and be clear about how you’re going to use it.
Implementing Responsible AI Practices
Responsible AI isn’t just a buzzword; it’s a way of thinking about AI development and deployment. It means considering the potential risks and benefits of your AI systems, and taking steps to minimize the risks. Here are some things to keep in mind:
- Establish clear ethical guidelines for your AI projects.
- Create a process for reviewing and auditing your AI systems.
- Train your team on responsible AI principles.
- Be prepared to explain your AI decisions to stakeholders.
It’s important to remember that AI is a tool, and like any tool, it can be used for good or for bad. It’s up to us to make sure that we’re using AI in a way that benefits society as a whole. This means being proactive about addressing ethical concerns and implementing responsible AI practices.
Here’s a simple table to illustrate the importance of responsible AI:
Aspect | Why It Matters |
---|---|
Fairness | Prevents discrimination and ensures equal opportunities. |
Transparency | Builds trust and allows for accountability. |
Privacy | Protects individuals’ rights and prevents misuse of personal information. |
Accountability | Ensures that someone is responsible for the AI’s actions. |
Human Oversight | Prevents AI from making decisions that could harm people. |
By focusing on these areas, you can build ethical guidelines into your AI strategy and make sure you’re using Relevance AI in a responsible and beneficial way.
Final Thoughts
In the end, a solid Data + AI strategy can really change the game for your business. It’s not just about keeping up; it’s about finding new ways to innovate and stay ahead. By aligning your goals, building a strong data foundation, and getting your team on board, you set yourself up for long-term success. With the right approach, you can tap into the power of data and AI to drive growth and agility. So, don’t wait too long—now’s the time to turn your ideas into real results.
Frequently Asked Questions
What is Relevance AI?
Relevance AI is a tool that helps businesses use data better by making AI smarter and more useful for decision-making.
How can Relevance AI help my business?
It can improve how you understand your data, align your AI tools with your business goals, and enhance your daily operations.
What are some examples of using Relevance AI?
You can use it to personalize customer experiences, improve workflows, and make data-driven decisions.
What should I consider when integrating Relevance AI into my company?
Look at how it can fit into your daily tasks, ensure your team is trained, and measure the results with clear goals.
How does Relevance AI affect customer engagement?
It allows businesses to create personalized experiences for customers, making interactions more relevant and satisfying.
What are the ethical concerns with using Relevance AI?
It’s important to ensure fairness, protect customer privacy, and use data responsibly when implementing AI solutions.